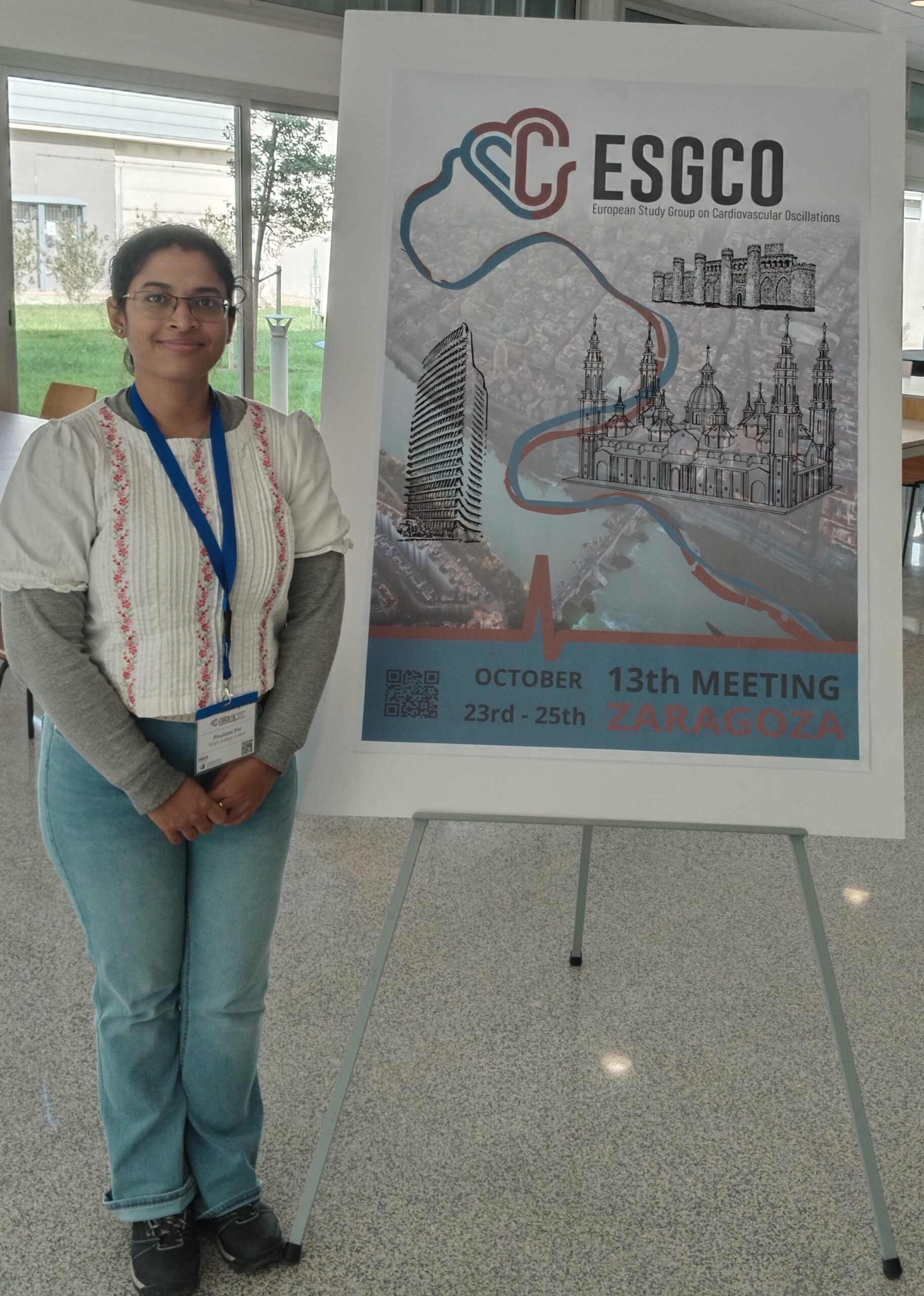
Poulomi Pal presents a Graphical Convolution Neural Network (GCNN) for music based hypertension diagnosis using ECG, respiration, and pulse signals at ESGCO, the European Study Group on Cardiovascular Oscillations in Zaragoza, Spain, on Thursday, 24 October 2024.
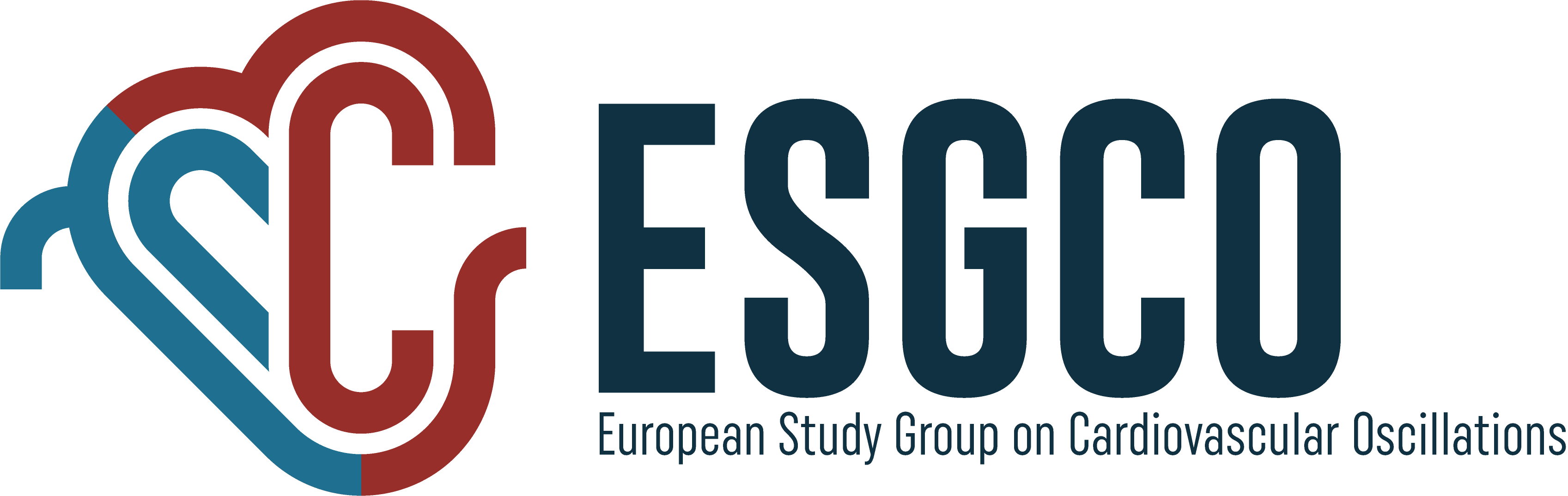
Results show music listening boosts hypertension diagnosis results (accuracy, recall, specificity, F1) by 10% over silence baseline.
Pal, P, N Cotic, M Soliński, V Pope, P Lambiase, E Chew (2024) Music-based Graph Convolution Neural Network with ECG, Respiration, Pulse Signal as a Diagnostic Tool for Hypertension. In Proceedings of the European Study Group in Cardiovascular Oscillations (ESGCO), Zaragoza, Spain, 23-25 Oct. [ preprint ]
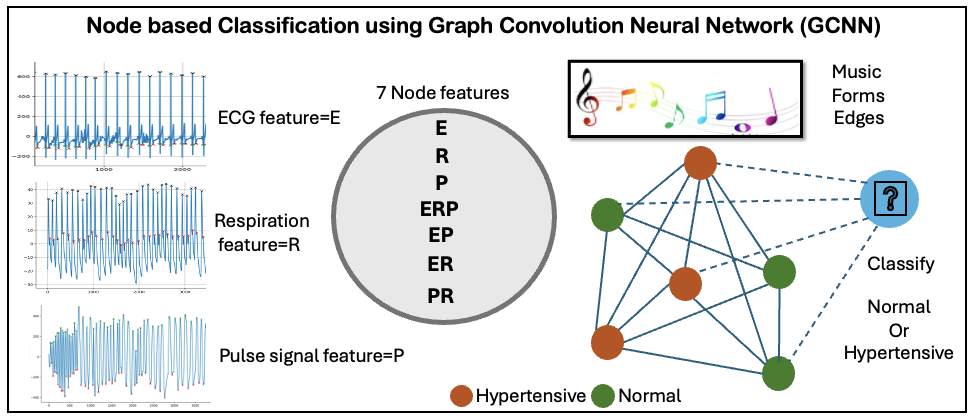
13th ESGCO (European Study Group on Cardiovascular Oscillations) meeting: Zaragoza (Spain) 23-25 Oct 2024
Music-based Graph Convolution Neural Network with ECG, Respiration, Pulse Signal as a Diagnostic Tool for Hypertension
Authors: Poulomi Pal, Natalia Cotic, Mateusz Solinski, Vanessa Pope, Pier Lambiase, and Elaine Chew
Thursday, 24 Oct 2024, 16:45 – 17:30
Abstract: Hypertension is one of the prime risk factors of cardiovascular disease. Music has been shown to be beneficial for lowering blood pressure. Here, we investigate if music can help in identifying hypertensive individuals. We acquire simultaneously electrocardiography (ECG), respiration, and pulse signals from 70 participants whilst they listen to music that has been altered digitally to differ only in tempi and loudness. Baseline blood pressure values in the preceding silence was taken as ground truth. After pre-processing, we obtain feature indices E, R, P from the ECG, respiration and pulse signals, respectively. The indices are fused to derive the compound indices ERP, EP, RP, and ER. Classification was performed using GCNN (Graph Convolution Neural Network) to segregate hypertensives from normotensive individuals. The index values formed the nodes, and the music attributes (average tempo and loudness) were used to establish the edge connectivity for node based classification. Binary classification was carried out with 0.85 accuracy, 0.87 recall, 0.84 specificity, and 0.86 F1-score. Without edges (music attributes), classification performance was 10% lower on average. We demonstrate for the first time the potential of music-based hypertension diagnosis using listeners’ ECG, respiration, and pulse signal during music.
Speaker: Poulomi Pal, King’s College London, Postdoctoral Research Associate
Session: Physiological networks (Session 6A)
Session Chair: Lucas Faes and Gonzalo C. Gutiérrez
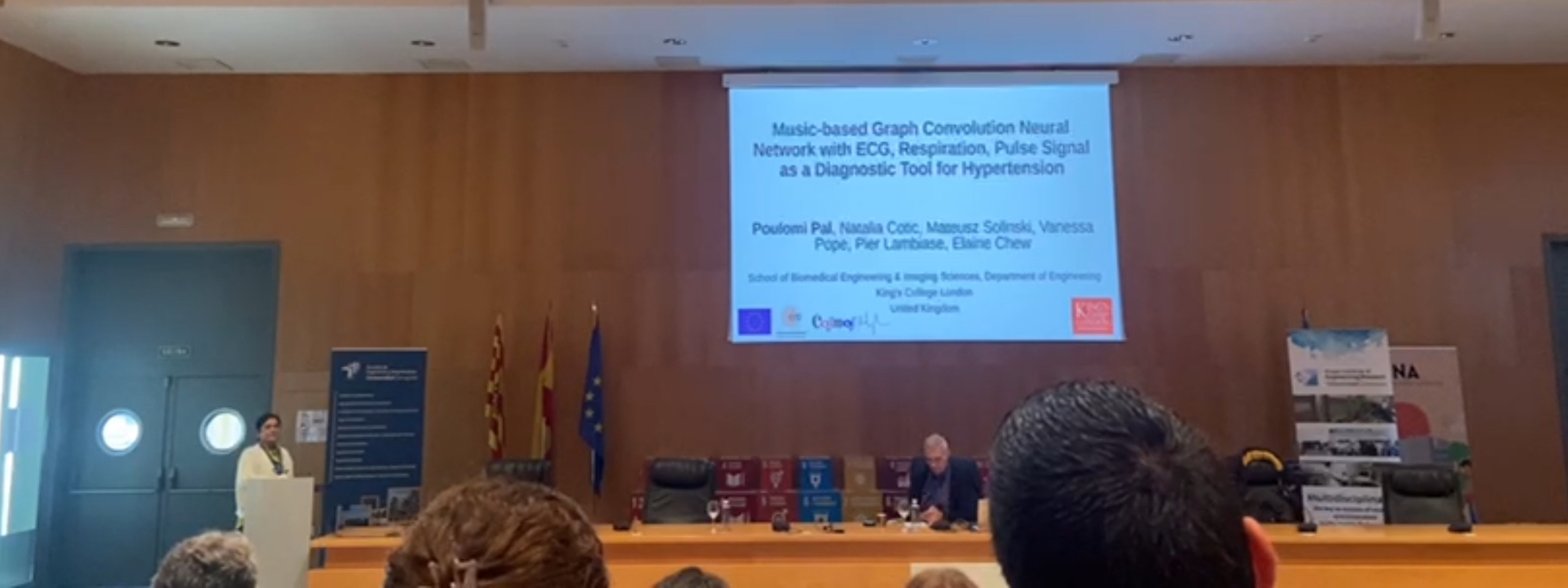
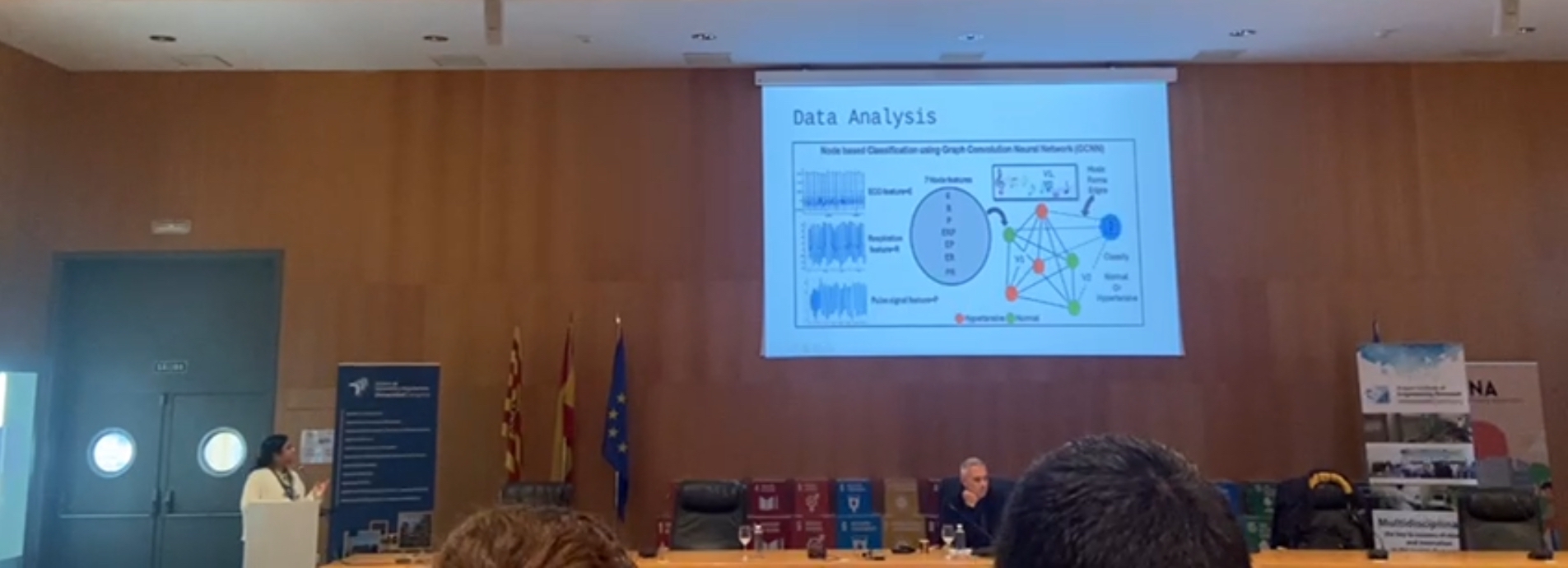
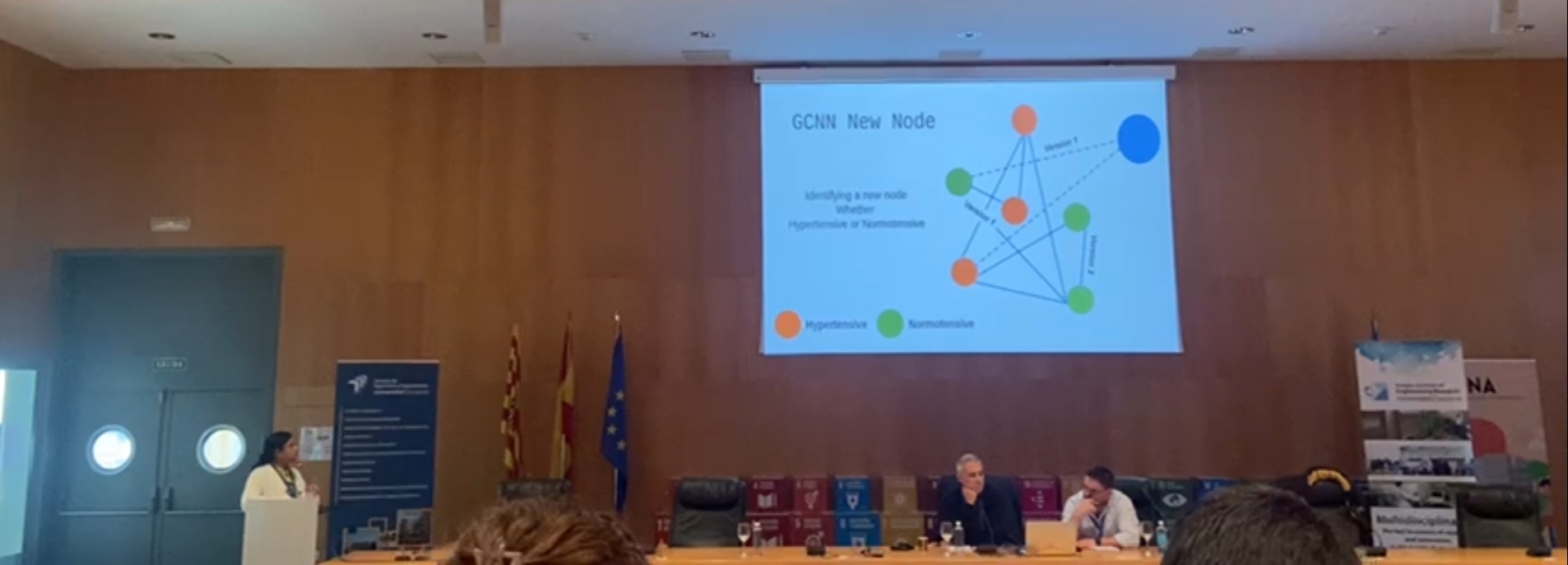